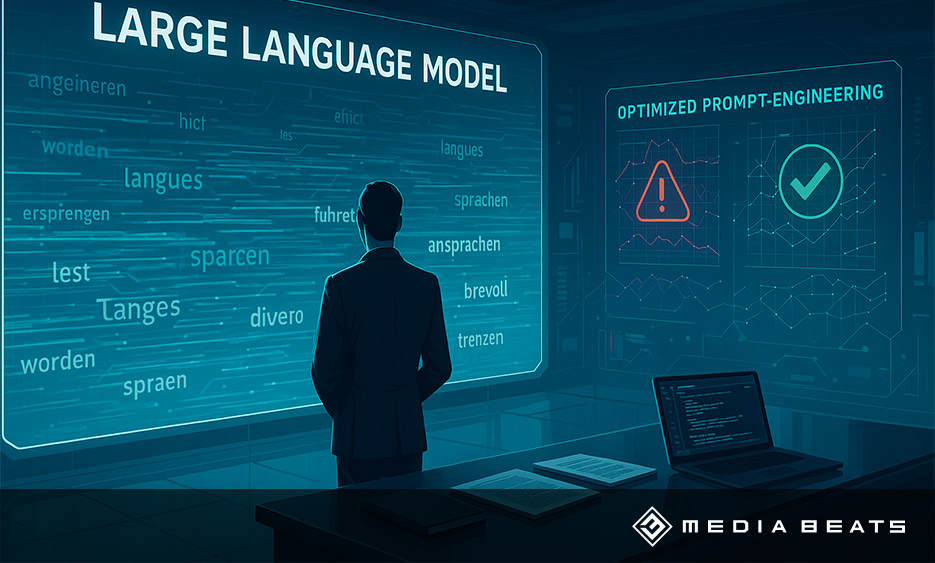
How prompt engineering reduces hallucinations in AI models
Hallucinations – meaning false or fabricated information – frequently occur in large language models like ChatGPT. But there is hope, as targeted prompt engineering can significantly reduce this weakness.
In this article, you’ll learn how current research and proven strategies can improve the performance of language models. We’ll also highlight the limitations of these methods in practice and what to watch out for.
What are hallucinations in language models?
Before we explain the techniques of prompt engineering, we first need to understand what hallucinations mean in this context. Language models like ChatGPT are based on probabilistic calculations and therefore sometimes produce false or fabricated information. This issue is particularly critical in fields such as medicine, law, and software development, where precise information is essential.
How prompt engineering helps to reduce hallucinations
The good news: Prompt engineering is highly effective and helps to significantly reduce the frequency of hallucinations. Numerous studies and experiments show that precise and context-rich inputs can greatly improve accuracy. A good example is the chain-of-thought technique, in which the model must respond logically in multiple steps. This not only leads to fewer errors but also results in significantly better coherence in the generated content. A recent study showed that this technique increased answer accuracy in math problems by 30 percent.
Best practices for optimizing prompts
A well-thought-out prompt strategy is essential for effectively reducing hallucinations. Here are some proven practical approaches:
1. Precision in the prompt
The more precise and specific the input, the higher the likelihood of receiving an accurate answer. Instead of simply asking, “Tell me something about history,” you should phrase it more specifically. A better question would be: “Describe the key events of the French Revolution in three sentences and cite a source.” This gives the model clear instructions, which significantly reduces the likelihood of speculation.
2. Put the model in a certain role
By assigning the model a specific expert role, both accuracy and style improve. An instruction like “You are a historian” prompts the model to respond in a more scholarly and precise manner. This technique is especially effective when combined with other methods, such as providing sources.
3. Step-by-step thinking (chain-of-thought)
Requiring step-by-step thinking forces the model to build its arguments in a logical and structured way. This avoids jumping to conclusions and stays closer to verifiable information. Especially with complex tasks, this method helps to reduce errors and achieve more precise results.
4. Retrieval of external data
The RAG method is particularly suitable if the model is to use external sources to support its answer. In this way, the model avoids unreliable assumptions and does not rely on outdated information. Instead, it integrates current and verified facts directly into the answer.
5. Self-checking and verification
Incorporating self-criticism into the response process is another helpful method for improving quality. If the model questions and checks its answer, it recognizes possible errors at an early stage. This technique significantly increases the accuracy of the results, especially in complex situations.
Application examples: Where hallucinations are particularly problematic
In many industries where precision is crucial, hallucinations can have serious consequences. This is especially evident in medicine, where false information can be dangerous. One study found that ChatGPT cited fabricated sources in up to 47 percent of scientific texts. Such errors can potentially endanger patient safety. That’s why it’s important to connect the model to reliable sources and involve human experts.
In the legal field as well, hallucinations pose a serious risk. In a well-known case, ChatGPT generated incorrect legal citations for a lawsuit. Targeted prompt engineering using legal sources can significantly improve the accuracy of responses.
Conclusion
In conclusion, prompt engineering is an effective tool against hallucinations in language models. Targeted inputs prompt the model to work more precisely and rely on trustworthy sources. Self-checking also helps to noticeably reduce the error rate. However, in safety-critical fields such as medicine or law, human oversight remains indispensable.
Would you like to know how to successfully use language models for your online marketing? Then contact us today! As a full-service agency, we develop customized solutions in performance marketing, email marketing, and content strategies, supporting you in achieving your goals with the latest technology.